PyTorch Features
PyTorch is an open-source machine learning library for Python that provides tensor computation with GPU acceleration and a dynamic computational graph.
View MoreKey Features of PyTorch
PyTorch is an open-source machine learning library that provides tensor computation with strong GPU acceleration, dynamic neural networks, and deep integration with Python. It offers a flexible ecosystem for building and deploying AI models, with features like eager execution, distributed training, robust production deployment tools, and extensive cloud platform support.
Dynamic Computational Graphs: Allows for dynamic definition and modification of neural network architectures during runtime, providing greater flexibility for complex models.
Native Python Integration: Seamlessly integrates with Python's data science stack, enabling users to leverage familiar tools and libraries in their workflows.
Distributed Training: Supports scalable distributed training across multiple GPUs and machines, enabling efficient training of large models on big datasets.
TorchScript and TorchServe: Provides tools for optimizing models for production deployment, including graph-based intermediate representation and serving infrastructure.
Extensive Ecosystem: Offers a rich set of tools, libraries, and frameworks that extend PyTorch's capabilities for various domains like computer vision and NLP.
Use Cases of PyTorch
Computer Vision: Building and training advanced image recognition, object detection, and segmentation models for applications in autonomous vehicles, medical imaging, and more.
Natural Language Processing: Developing state-of-the-art language models, machine translation systems, and conversational AI using PyTorch's flexible neural network architectures.
Scientific Computing: Leveraging PyTorch's numerical computing capabilities and GPU acceleration for simulations, data analysis, and modeling in physics, chemistry, and other scientific domains.
Recommender Systems: Creating personalized recommendation engines for e-commerce, content platforms, and social media using PyTorch's deep learning capabilities.
Pros
Intuitive and Pythonic API making it easy to learn and use
Dynamic computation graphs allowing for flexible model architectures
Strong community support and extensive ecosystem of tools and libraries
Excellent performance and GPU acceleration capabilities
Cons
Slightly steeper learning curve compared to some other frameworks for beginners
Smaller ecosystem compared to TensorFlow, though rapidly growing
Can be more memory-intensive than static graph frameworks in some cases
PyTorch Monthly Traffic Trends
PyTorch experienced a -8.7% decline in traffic, possibly due to ongoing bug issues such as the MPS backend memory leak and flex attention crashes with torch.amp. Additionally, the announcement that TorchServe is no longer actively maintained might have raised concerns among developers about the future of PyTorch in production environments.
View history traffic
Related Articles
Popular Articles
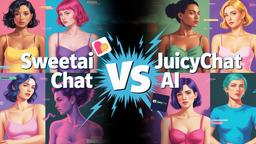
SweetAI Chat VS JuicyChat AI: Why SweetAI Chat Wins in 2025
Jun 18, 2025
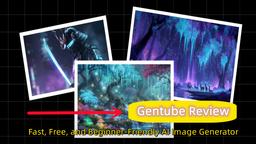
Gentube Review 2025: Fast, Free, and Beginner-Friendly AI Image Generator
Jun 16, 2025
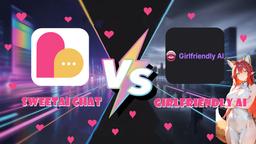
SweetAI Chat vs Girlfriendly AI: Why SweetAI Chat Is the Better Choice in 2025
Jun 10, 2025
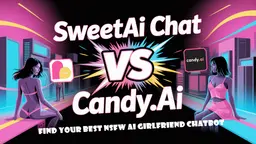
SweetAI Chat vs Candy.ai 2025: Find Your Best NSFW AI Girlfriend Chatbot
Jun 10, 2025
View More