Cerebras Features
Cerebras Systems adalah perusahaan komputasi AI perintis yang membangun prosesor AI terbesar dan tercepat di dunia - Wafer Scale Engine (WSE) - yang dirancang untuk mempercepat pelatihan dan beban kerja inferensi AI.
Lihat Lebih BanyakFitur Utama Cerebras
Cerebras adalah perusahaan komputasi AI perintis yang mengembangkan chip AI terbesar di dunia (Wafer Scale Engine) dan superkomputer AI. Teknologi mereka menawarkan kinerja revolusioner untuk pelatihan dan inferensi AI, dengan bandwidth memori yang belum pernah ada sebelumnya, penyimpanan terintegrasi di chip, dan arsitektur khusus untuk beban kerja AI. Chip WSE-3 terbaru perusahaan memberikan kecepatan dan efisiensi superior dibandingkan solusi GPU tradisional, mampu menangani model dengan hingga 24 triliun parameter.
Wafer Scale Engine (WSE): Chip komputer terbesar di dunia, seukuran piring makan, dengan 44GB SRAM di chip dan 900.000 inti komputasi, memungkinkan seluruh model AI disimpan langsung di chip
Inferensi Berkecepatan Tinggi: Menghasilkan hingga 2100 token/detik untuk inferensi LLM, yang 68x lebih cepat daripada solusi cloud berbasis GPU, dengan sepertiga biaya dan sepertiga konsumsi daya
Arsitektur Memori Terintegrasi: Memiliki bandwidth memori agregat 21 petabyte/detik (7000x dari Nvidia H100), menghilangkan hambatan memori tradisional dalam pemrosesan AI
Model Pemrograman Sederhana: Menghilangkan kebutuhan untuk pemrograman terdistribusi yang kompleks dan manajemen kluster melalui platform perangkat lunak terintegrasi yang kompatibel dengan TensorFlow dan PyTorch
Kasus Penggunaan Cerebras
Pelatihan Model Bahasa Besar: Mempercepat pelatihan model bahasa besar dari bulan menjadi jam, mendukung model dengan miliaran hingga triliunan parameter
Pengembangan AI Kesehatan: Bermitra dengan institusi seperti Mayo Clinic untuk mengembangkan model AI khusus untuk aplikasi dan penelitian kesehatan
Komputasi Berkinerja Tinggi: Memberdayakan pusat superkomputasi dan fasilitas penelitian untuk komputasi ilmiah dan simulasi kompleks
Inferensi AI Perusahaan: Menyediakan layanan inferensi berkecepatan tinggi dan biaya efektif untuk bisnis yang menerapkan model AI besar di lingkungan produksi
Kelebihan
Kecepatan pemrosesan dan efisiensi yang belum pernah ada sebelumnya untuk beban kerja AI
Pemrograman dan penerapan yang disederhanakan dibandingkan dengan sistem terdistribusi
Bandwidth memori superior dan arsitektur terintegrasi
Kekurangan
Biaya investasi awal yang tinggi
Ketergantungan berat pada satu pelanggan (G42) untuk pendapatan
Teknologi yang relatif baru dengan rekam jejak terbatas dibandingkan solusi yang sudah mapan
Tren Traffic Bulanan Cerebras
Cerebras mencapai 600 ribu kunjungan dengan pertumbuhan 35,0% dalam kunjungan bulanan. Peluncuran enam pusat data inferensi AI baru dan DeepSeek R1-70B tercepat di dunia dengan kecepatan lebih dari 1500 token per detik kemungkinan berkontribusi pada peningkatan lalu lintas.
Lihat riwayat traffic
Artikel Terkait
Artikel Populer
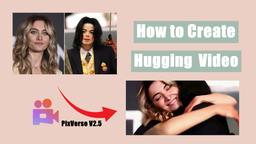
Tutorial Video Berpelukan PixVerse V2.5 | Cara Membuat Video Berpelukan AI di Tahun 2025
Apr 22, 2025
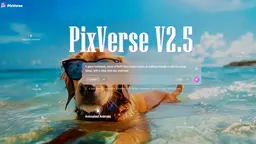
Rilis PixVerse V2.5: Ciptakan Video AI Tanpa Cela Tanpa Lag atau Distorsi!
Apr 21, 2025
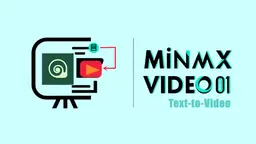
MiniMax Video-01(Hailuo AI): Lompatan Revolusioner AI dalam Pembuatan Teks-ke-Video 2025
Apr 21, 2025
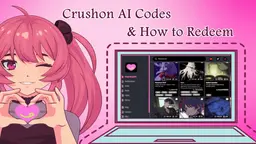
Kode Hadiah Baru CrushOn AI NSFW Chatbot di Bulan April 2025 dan Cara Menukarkannya
Apr 21, 2025
Lihat Selengkapnya