Tensorfuse Özellikler
Tensorfuse, kendi bulut altyapınızda üretken AI modellerinin kolay dağıtımını ve otomatik ölçeklenmesini sağlayan sunucusuz bir GPU platformudur.
Daha Fazla GösterTensorfuse Temel Özellikleri
Tensorfuse, kullanıcıların kendi bulut altyapılarında üretken AI modellerini dağıtmasına ve otomatik ölçeklendirmesine olanak tanıyan sunucusuz bir GPU platformudur. Dağıtım için basit bir CLI arayüzü, trafiğe yanıt olarak otomatik ölçeklendirme ve AWS, Azure ve GCP gibi büyük bulut sağlayıcılarıyla uyumluluk sağlar. Tensorfuse, kullanıcıların özel bulutlarında verileri ve modelleri tutarken, özelleştirilebilir ortamlar, OpenAI uyumlu uç noktalar ve maliyet etkin kaynak kullanımı gibi özellikler sunar.
Sunucusuz GPU Dağıtımı: Basit bir CLI arayüzü kullanarak kendi bulut altyapınızda üretken AI modellerini dağıtın ve otomatik ölçeklendirin.
Çoklu Bulut Uyumluluğu: AWS, Azure ve GCP gibi büyük bulut sağlayıcılarını destekleyerek platformlar arasında hesaplama kaynaklarının esnek kullanımına olanak tanır.
Özelleştirilebilir Ortamlar: Karmaşık YAML yapılandırmalarına ihtiyaç duymadan, basit Python kodu kullanarak konteyner görüntülerini ve donanım spesifikasyonlarını tanımlayın.
OpenAI Uyumlu API: Mevcut uygulamalar ve iş akışlarıyla kolay entegrasyon için OpenAI uyumlu bir uç nokta sağlar.
Özel Bulut Dağıtımı: Modelleri ve verileri kullanıcının özel bulut ortamında tutarak veri gizliliği ve güvenliği sağlar.
Tensorfuse Kullanım Alanları
Düzenlemelere Tabi Sektörler için AI Model Dağıtımı: Finansal kurumlar veya sağlık hizmeti sağlayıcıları, veri gizliliği düzenlemelerine uyumu sağlamak için AI modellerini kendi altyapılarında dağıtabilir.
Ölçeklenebilir NLP Hizmetleri: Doğal dil işleme hizmetleri sunan şirketler, sunucuları yönetmeden değişen talebi karşılamak için altyapılarını kolayca ölçeklendirebilir.
Maliyet Etkin Makine Öğrenimi Araştırması: Araştırma kurumları, hesaplama ihtiyaçlarına göre yukarı veya aşağı ölçeklendirerek GPU kaynaklarını verimli bir şekilde kullanabilir, boşta kalma süresini ve maliyetleri azaltabilir.
Çoklu Bulut AI Stratejisi: Şirketler, AI iş yükleri için çoklu bulut stratejisi uygulayarak modelleri farklı bulut sağlayıcıları arasında dağıtarak optimal performans ve yedeklilik sağlayabilir.
Artıları
Özel bulut altyapısında AI modellerinin dağıtımını ve ölçeklendirilmesini basitleştirir
Kullanıma dayalı model ile maliyet etkin kaynak kullanımı sunar
Modelleri ve verileri kullanıcının bulutunda tutarak veri gizliliği ve güvenliği sağlar
Eksileri
Kurulum ve yapılandırma için bazı teknik uzmanlık gerektirebilir
Desteklenen bulut sağlayıcılarıyla sınırlıdır (AWS, Azure, GCP)
Bulut sağlayıcı ücretlerinin üzerine ek hesaplama yönetim maliyetleri
Tensorfuse Aylık Trafik Trendleri
Tensorfuse geçen ay 9.8k ziyaret aldı ve -63.4% oranında bir Önemli Düşüş gösterdi. Analizimize göre, bu eğilim yapay zeka araçları sektöründeki tipik pazar dinamikleriyle uyumludur.
Geçmiş trafiği görüntüle
Popüler Makaleler
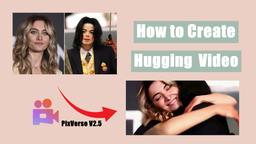
PixVerse V2.5 Sarılma Videosu Eğitimi | 2025'te Yapay Zeka Sarılma Videoları Nasıl Oluşturulur
Apr 22, 2025
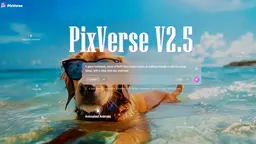
PixVerse V2.5 Sürümü Yayınlandı: Hatasız, Gecikmesiz veya Bozulmasız Yapay Zeka Videoları Oluşturun!
Apr 21, 2025
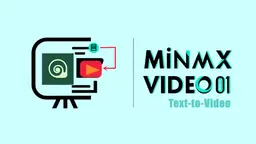
MiniMax Video-01(Hailuo AI): Yapay Zekanın Metinden Videoya Dönüştürmede Devrim Niteliğindeki Atılımı 2025
Apr 21, 2025
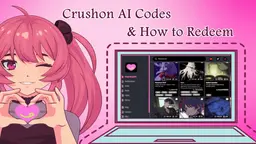
CrushOn AI NSFW Chatbot Nisan 2025'te Yeni Hediye Kodları ve Nasıl Kullanılır
Apr 21, 2025
Daha Fazla Göster