Captum · Model Interpretability for PyTorch Funktionen
WebsiteAI Data Mining
Captum ist eine Open-Source-Bibliothek zur Modellinterpretierbarkeit für PyTorch, die multimodale Modelle unterstützt und modernste Attributionsalgorithmen bereitstellt.
Mehr anzeigenHauptfunktionen von Captum · Model Interpretability for PyTorch
Captum ist eine Open-Source-Bibliothek zur Interpretierbarkeit von Modellen für PyTorch, die modernste Algorithmen bereitstellt, um Forschern und Entwicklern zu helfen, zu verstehen, welche Merkmale zu den Vorhersagen eines Modells beitragen. Es unterstützt die Interpretierbarkeit über verschiedene Modalitäten hinweg, einschließlich Vision und Text, funktioniert mit den meisten PyTorch-Modellen und bietet ein erweiterbares Framework zur Implementierung neuer Interpretierbarkeitsalgorithmen.
Multi-Modal Unterstützung: Unterstützt die Interpretierbarkeit von Modellen über verschiedene Modalitäten hinweg, einschließlich Vision, Text und mehr.
PyTorch-Integration: Basierend auf PyTorch und unterstützt die meisten Arten von PyTorch-Modellen mit minimalen Änderungen am ursprünglichen neuronalen Netzwerk.
Erweiterbares Framework: Open-Source, generische Bibliothek, die eine einfache Implementierung und Benchmarking neuer Interpretierbarkeitsalgorithmen ermöglicht.
Umfassende Attributionsmethoden: Bietet verschiedene Attributionsalgorithmen, einschließlich integrierter Gradienten, Salienz-Maps und TCAV, um die Merkmalsbedeutung zu verstehen.
Visualisierungstools: Bietet Captum Insights, ein interaktives Visualisierungs-Widget für das Debugging von Modellen und die Visualisierung der Merkmalsbedeutung.
Anwendungsfälle von Captum · Model Interpretability for PyTorch
Verbesserung der Modellleistung: Forscher und Entwickler können Captum verwenden, um zu verstehen, welche Merkmale zu den Vorhersagen des Modells beitragen, und ihre Modelle entsprechend zu optimieren.
Debugging von Deep-Learning-Modellen: Captum kann verwendet werden, um die inneren Abläufe komplexer Deep-Learning-Modelle zu visualisieren und zu verstehen, was beim Debugging und der Verfeinerung hilft.
Sicherstellung der Fairness von Modellen: Durch das Verständnis der Merkmalsbedeutung kann Captum helfen, Vorurteile in maschinellen Lernmodellen in verschiedenen Branchen zu identifizieren und zu mindern.
Verbesserung der erklärbaren KI im Gesundheitswesen: Medizinische Fachkräfte können Captum verwenden, um die Entscheidungen von KI-Modellen in Diagnosen oder Behandlungsempfehlungen zu interpretieren, was Vertrauen und Transparenz erhöht.
Vorteile
Umfassende Sammlung von Interpretierbarkeitsalgorithmen
Nahtlose Integration mit PyTorch
Unterstützt multi-modale Interpretierbarkeit
Open-Source und erweiterbar
Nachteile
Begrenzt auf PyTorch-Modelle
Kann ein tiefes Verständnis der Konzepte zur Interpretierbarkeit für eine effektive Nutzung erfordern
Captum · Model Interpretability for PyTorch Monatliche Traffic-Trends
Captum · Model Interpretability for PyTorch erhielt im letzten Monat 17.0k Besuche, was ein Leichtes Wachstum von 16.4% zeigt. Basierend auf unserer Analyse entspricht dieser Trend der typischen Marktdynamik im Bereich der KI-Tools.
Verlaufsdaten anzeigen
Beliebte Artikel
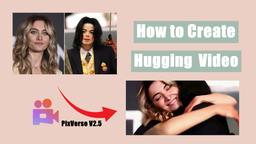
PixVerse V2.5 Tutorial für Umarmungsvideos | So erstellen Sie KI-Umarmungsvideos im Jahr 2025
Apr 22, 2025
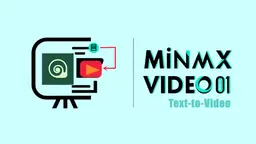
MiniMax Video-01(Hailuo AI): Revolutionärer KI-Sprung in der Text-zu-Video-Generierung 2025
Apr 21, 2025
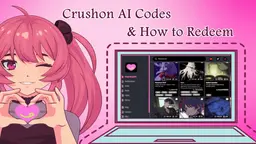
CrushOn AI NSFW Chatbot: Neue Geschenkcodes im April 2025 und wie man sie einlöst
Apr 21, 2025
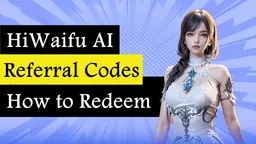
HiWaifu AI Empfehlungscodes im April 2025 und wie man sie einlöst
Apr 21, 2025
Mehr anzeigen