Collaborative Language Model Runner
Petals is an open-source system that enables collaborative inference and fine-tuning of large language models by distributing model parts across multiple users.
https://petals.ml/
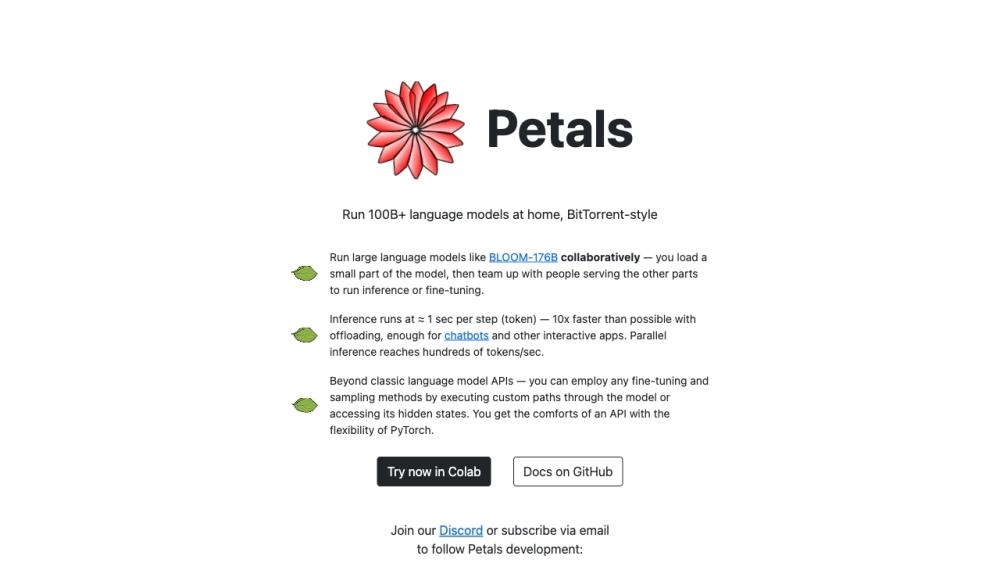
Product Information
Updated:Dec 16, 2024
What is Collaborative Language Model Runner
Petals is an innovative framework that allows users to run and fine-tune large language models (LLMs) with over 100 billion parameters collaboratively. Developed as part of the BigScience project, Petals aims to democratize access to powerful LLMs like BLOOM-176B by creating a decentralized network where users can contribute their computational resources. This system overcomes the hardware limitations that typically prevent individual researchers from utilizing such massive models, making advanced NLP capabilities more accessible to a wider audience.
Key Features of Collaborative Language Model Runner
Petals is an open-source decentralized system that enables collaborative inference and fine-tuning of large language models (LLMs) with over 100 billion parameters. It allows users to run these models by loading only a small part locally and teaming up with others serving the remaining parts, making LLMs accessible without high-end hardware requirements.
Distributed Model Execution: Runs large language models by splitting them across multiple machines in a BitTorrent-style network.
Flexible API: Provides a PyTorch-based API that allows custom fine-tuning, sampling methods, and access to model internals.
Efficient Inference: Enables inference up to 10x faster than traditional offloading techniques.
Collaborative Fine-tuning: Allows users to fine-tune large models collaboratively using distributed resources.
Use Cases of Collaborative Language Model Runner
Research and Experimentation: Enables researchers to experiment with large language models without expensive hardware.
Interactive AI Applications: Supports building interactive AI applications like chatbots with reduced latency.
Democratized AI Access: Makes powerful language models accessible to a wider range of users and organizations.
Custom Model Adaptation: Allows fine-tuning of large models for specific domains or tasks collaboratively.
Pros
Reduces hardware costs for using large language models
Enables flexible research and experimentation
Improves inference speed compared to offloading
Cons
Relies on community participation and resource sharing
May have privacy concerns when processing sensitive data
Performance depends on network conditions and available peers
How to Use Collaborative Language Model Runner
Install Petals: Install Petals and its dependencies using pip: pip install git+https://github.com/bigscience-workshop/petals
Import required modules: Import the necessary modules from Petals and Transformers: from transformers import AutoTokenizer; from petals import AutoDistributedModelForCausalLM
Choose a model: Select a large language model available on the Petals network, such as 'meta-llama/Meta-Llama-3.1-405B-Instruct'
Initialize tokenizer and model: Create the tokenizer and model objects: tokenizer = AutoTokenizer.from_pretrained(model_name); model = AutoDistributedModelForCausalLM.from_pretrained(model_name)
Prepare input: Tokenize your input text: inputs = tokenizer(prompt, return_tensors='pt')
Generate output: Use the model to generate text based on the input: outputs = model.generate(**inputs, max_new_tokens=100)
Decode output: Decode the generated token IDs back into text: generated_text = tokenizer.decode(outputs[0])
Optional: Contribute resources: To help expand the network, you can run a Petals server to share your GPU: python -m petals.cli.run_server model_name
Collaborative Language Model Runner FAQs
Petals is an open-source system that allows users to run large language models (100B+ parameters) collaboratively in a distributed manner, similar to BitTorrent. It enables running models like BLOOM-176B for inference and fine-tuning by having users load small parts of the model and teaming up with others.
Analytics of Collaborative Language Model Runner Website
Collaborative Language Model Runner Traffic & Rankings
0
Monthly Visits
-
Global Rank
-
Category Rank
Traffic Trends: May 2024-Jan 2025
Collaborative Language Model Runner User Insights
-
Avg. Visit Duration
0
Pages Per Visit
0%
User Bounce Rate
Top Regions of Collaborative Language Model Runner
Others: 100%