Lantern Features
Lantern is an open-source PostgreSQL vector database extension that provides high-performance vector search capabilities for building AI applications.
View MoreKey Features of Lantern
Lantern is a powerful PostgreSQL vector database extension designed for building AI applications. It offers fast vector indexing, efficient search capabilities, and easy embedding generation. Lantern provides a managed cloud service as well as self-hosting options, allowing developers to leverage vector search within their existing Postgres databases. With features like one-click vector generation, support for multiple embedding models, and cost-effective scalability, Lantern aims to simplify the development of AI-powered applications.
Fast Vector Indexing: Lantern's index creation is 30x faster than pgvector, enabling rapid setup of vector search capabilities.
One-Click Embedding Generation: Easily generate vector embeddings from unstructured data using over 20 supported embedding models with a single click.
Cost-Effective Scalability: Lantern offers high performance at a fraction of the cost compared to standalone vector databases, potentially saving up to 94% on cloud costs.
SQL and ORM Integration: Perform vector operations using familiar SQL queries or popular ORM libraries, simplifying integration with existing applications.
Managed Cloud Service: Lantern Cloud provides a fully managed database offering with support for embedding generation and management.
Use Cases of Lantern
AI-Powered Search Systems: Implement semantic search in applications by leveraging vector embeddings to find similar content or documents.
Recommendation Engines: Build personalized recommendation systems using vector similarity to suggest products, content, or services to users.
Natural Language Processing Applications: Develop chatbots, text classification, or sentiment analysis tools using vector representations of text data.
Image and Video Analysis: Create systems for image recognition, visual search, or content-based video retrieval using vector embeddings of visual data.
Fraud Detection: Implement anomaly detection systems in financial services by comparing transaction patterns using vector similarity.
Pros
Integrates seamlessly with existing PostgreSQL databases
Offers significant cost savings compared to standalone vector databases
Provides both managed cloud and self-hosted options for flexibility
Supports a wide range of embedding models and easy vector generation
Cons
Relatively new product, may have less community support than more established solutions
Limited to PostgreSQL environments, not suitable for users of other database systems
May require some learning curve for developers not familiar with vector databases
Popular Articles
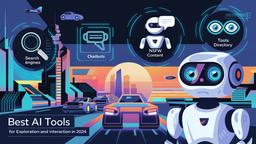
Best AI Tools for Exploration and Interaction in 2024: Search Engines, Chatbots, NSFW Content, and Comprehensive Directories
Dec 11, 2024
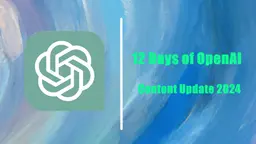
12 Days of OpenAI Content Update 2024
Dec 11, 2024
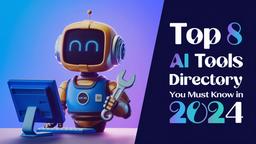
Top 8 AI Tools Directory in December 2024
Dec 11, 2024
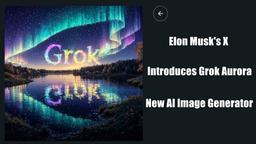
Elon Musk's X Introduces Grok Aurora: A New AI Image Generator
Dec 10, 2024
View More