AI hallucinations, where generative AI models produce incorrect or misleading information, have become a significant challenge in the field of artificial intelligence. Despite advancements, these inaccuracies can undermine trust and have serious real-world implications. This article delves into the causes of AI hallucinations and explores the latest developments and strategies to mitigate them.
What Causes AI Hallucinations?
AI hallucinations occur when AI models generate outputs that are not based on their training data or logical patterns. Several factors contribute to this phenomenon:
- Insufficient or Biased Training Data: AI models rely heavily on the quality of their training data. Insufficient, outdated, or biased data can lead to inaccurate outputs.
- Overfitting: When models are trained on limited datasets, they may memorize the data rather than generalize from it, leading to hallucinations.
- Complexity and Ambiguity: High model complexity and ambiguous prompts can confuse AI models, resulting in nonsensical outputs.
- Adversarial Attacks: Deliberate manipulation of input data can trick AI models into producing incorrect responses.
Real-World Implications
AI hallucinations have led to several notable incidents:
- Legal Missteps: A US lawyer was fined for using ChatGPT, which fabricated non-existent legal cases in a court brief.
- Customer Service Errors: Air Canada faced legal issues when its chatbot incorrectly offered a discount, leading to a tribunal ruling against the airline.
- Misinformation Spread: Google's Bard chatbot falsely claimed that the James Webb Space Telescope had captured the first images of an exoplanet.
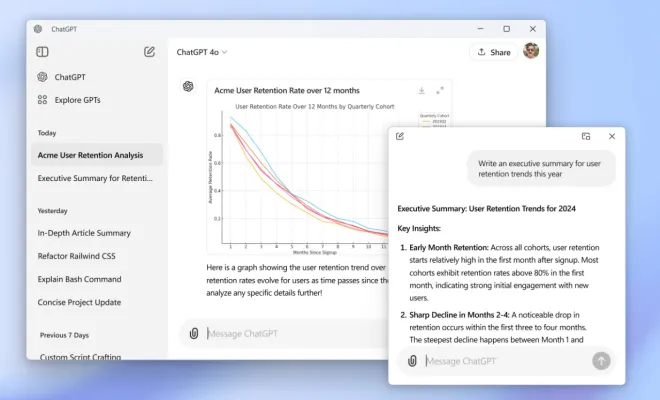
Mitigation Strategies
Efforts to reduce AI hallucinations focus on improving data quality, refining model training, and incorporating human oversight:
- High-Quality Training Data: Ensuring AI models are trained on diverse, balanced, and well-structured data helps minimize biases and inaccuracies.
- Retrieval Augmentation Generation (RAG): This technique improves AI model performance by retrieving relevant information from reliable sources before generating responses.
- Human Review Layers: Incorporating human fact-checkers to review AI outputs can catch and correct inaccuracies, enhancing the reliability of AI systems.
- Advanced Detection Algorithms: New algorithms are being developed to detect when AI models are likely to hallucinate, improving the accuracy of their outputs.
Future Directions
While significant progress has been made, AI hallucinations remain a challenge. Researchers are continuously developing new techniques to enhance AI reliability. For instance, blending technologies like intent identifiers, call classifiers, and sentiment analyzers with large language models (LLMs) can provide more accurate and contextually relevant responses.
As AI continues to evolve, it is crucial to address these challenges to fully realize the potential of generative AI. By improving data quality, refining training processes, and incorporating robust oversight mechanisms, we can mitigate the risks associated with AI hallucinations.
For more insights into AI advancements and tools, visit AIPURE for comprehensive information and resources on the latest in artificial intelligence.